Weighted Fuzzy Dempster-Shafer Framework for Multimodal Information Integration
Article Type
Research Article
Publication Title
IEEE Transactions on Fuzzy Systems
Abstract
This study proposes an architecture based on a weighted fuzzy Dempster-Shafer framework (WFDSF), which can adjust weights associated with inconsistent evidence obtained by different classification approaches, to realize a fusion system for integrating multimodal information. The Dempster-Shafer theory (D-S theory) of evidence enables us to integrate heterogeneous information from multiple sources to obtain collaborative inferences for a given problem. To conquer various uncertainties associated with the collected information, our system assigns beliefs and plausibilities to possible hypotheses of each decision maker and uses a combination rule to fuse multimodal information. For information fusion, an important step in D-S aggregation is to find an appropriate basic probability assignment scheme for allocating support to each possible hypothesis/class, which remains an arduous and unsolved problem. Here, we propose a mathematical structure to aggregate weighted evidence extracted from two different types of approaches: fuzzy Naïve Bayes and nearest mean classification rule. Further, an intuitionistic belief assignment is employed to address uncertainties between hypotheses/classes. Finally, 12 benchmark problems from the UCI machine learning repository for classification are employed to validate the proposed WFDSF-based scheme. In addition, an application of WFDSF to a practical brain-computer interface problem involving multimodal data fusion is demonstrated in this study. The experimental results show that the WFDSF is superior to several existing methods.
First Page
338
Last Page
352
DOI
10.1109/TFUZZ.2017.2659764
Publication Date
2-1-2018
Recommended Citation
Liu, Yu Ting; Pal, Nikhil R.; Marathe, Amar R.; and Lin, Chin Teng, "Weighted Fuzzy Dempster-Shafer Framework for Multimodal Information Integration" (2018). Journal Articles. 1492.
https://digitalcommons.isical.ac.in/journal-articles/1492
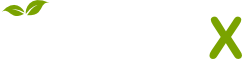
- Citations
- Citation Indexes: 79
- Policy Citations: 1
- Usage
- Abstract Views: 3
- Captures
- Readers: 48
Comments
All Open Access, Green