Minority Oversampling in Kernel Adaptive Subspaces for Class Imbalanced Datasets
Article Type
Research Article
Publication Title
IEEE Transactions on Knowledge and Data Engineering
Abstract
The class imbalance problem in machine learning occurs when certain classes are underrepresented relative to the others, leading to a learning bias toward the majority classes. To cope with the skewed class distribution, many learning methods featuring minority oversampling have been proposed, which are proved to be effective. To reduce information loss during feature space projection, this study proposes a novel oversampling algorithm, named minority oversampling in kernel adaptive subspaces (MOKAS), which exploits the invariant feature extraction capability of a kernel version of the adaptive subspace self-organizing maps. The synthetic instances are generated from well-trained subspaces and then their pre-images are reconstructed in the input space. Additionally, these instances characterize nonlinear structures present in the minority class data distribution and help the learning algorithms to counterbalance the skewed class distribution in a desirable manner. Experimental results on both real and synthetic data show that the proposed MOKAS is capable of modeling complex data distribution and outperforms a set of state-of-the-art oversampling algorithms.
First Page
950
Last Page
962
DOI
10.1109/TKDE.2017.2779849
Publication Date
5-1-2018
Recommended Citation
Lin, Chin Teng; Hsieh, Tsung Yu; Liu, Yu Ting; Lin, Yang Yin; Fang, Chieh Ning; Wang, Yu Kai; Yen, Gary; Pal, Nikhil R.; and Chuang, Chun Hsiang, "Minority Oversampling in Kernel Adaptive Subspaces for Class Imbalanced Datasets" (2018). Journal Articles. 1408.
https://digitalcommons.isical.ac.in/journal-articles/1408
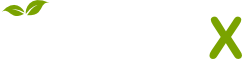
- Citations
- Citation Indexes: 58
- Policy Citations: 1
- Usage
- Abstract Views: 1
- Captures
- Readers: 46
Comments
All Open Access, Green