Machine learning detects EEG microstate alterations in patients living with temporal lobe epilepsy
Article Type
Research Article
Publication Title
Seizure
Abstract
Purpose: Quasi-stable electrical distribution in EEG called microstates could carry useful information on the dynamics of large scale brain networks. Using machine learning techniques we explored if abnormalities in microstates can identify patients with Temporal Lobe Epilepsy (TLE) in the absence of an interictal discharge (IED). Method: 4 Classes of microstates were computed from 2 min artefact free EEG epochs in 42 subjects (21 TLE and 21 controls). The percentage of time coverage, frequency of occurrence and duration for each of these microstates were computed and redundancy reduced using feature selection methods. Subsequently, Fishers Linear Discriminant Analysis (FLDA) and logistic regression were used for classification. Result: FLDA distinguished TLE with 76.1% accuracy (85.0% sensitivity, 66.6% specificity) considering frequency of occurrence and percentage of time coverage of microstate C as features. Conclusion: Microstate alterations are present in patients with TLE. This feature might be useful in the diagnosis of epilepsy even in the absence of an IED.
First Page
8
Last Page
13
DOI
10.1016/j.seizure.2018.07.007
Publication Date
10-1-2018
Recommended Citation
V, Kiran Raj; Rajagopalan, Shyam Sundar; Bhardwaj, Sujas; Panda, Rajanikant; Reddam, Venkateswara Reddy; Ganne, Chaitanya; Kenchaiah, Raghavendra; Mundlamuri, Ravindranadh C.; Kandavel, Thennarasu; Majumdar, Kaushik K.; Parthasarathy, Satishchandra; Sinha, Sanjib; and Bharath, Rose Dawn, "Machine learning detects EEG microstate alterations in patients living with temporal lobe epilepsy" (2018). Journal Articles. 1214.
https://digitalcommons.isical.ac.in/journal-articles/1214
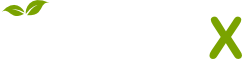
- Citations
- Citation Indexes: 51
- Policy Citations: 1
- Usage
- Abstract Views: 2
- Captures
- Readers: 180
Comments
All Open Access, Bronze, Green