Turning video into traffic data - An application to urban intersection analysis using transfer learning
Article Type
Research Article
Publication Title
IET Image Processing
Abstract
With modern socio-economic development, the number of vehicles in metropolitan cities is growing rapidly. Therefore, obtaining real-time traffic volume estimates has a very important significance in using the limited road space and traffic infrastructure. In this study, the authors present a video-based traffic volume and direction estimation at road intersections. To discriminate the vehicles from the remaining foreground objects, vehicle recognition is performed by training a deep-learning architecture from a pre-trained model. This method, called transfer learning, primarily circumvents the requirement of huge labelled datasets and the time for training the network. The video sequence is first detected for moving foreground regions or patches. The trained model is subsequently used to classify the vehicles. The vehicles are tracked, and trajectory patterns are clustered using standard techniques. The number and direction of vehicles are noted, which are later compared with the manually observed values. All experiments were performed on real-life surveillance sequences recorded at four different traffic intersections in the city of Kolkata.
First Page
673
Last Page
679
DOI
10.1049/iet-ipr.2018.5985
Publication Date
3-28-2019
Recommended Citation
Dey, Bhaskar and Kundu, Malay Kumar, "Turning video into traffic data - An application to urban intersection analysis using transfer learning" (2019). Journal Articles. 908.
https://digitalcommons.isical.ac.in/journal-articles/908
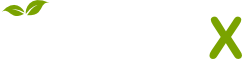
- Citations
- Citation Indexes: 20
- Policy Citations: 1
- Usage
- Abstract Views: 7
- Captures
- Readers: 31