Integration of deep feature extraction and ensemble learning for outlier detection
Article Type
Research Article
Publication Title
Pattern Recognition
Abstract
It is obvious to see that most of the datasets do not have exactly equal number of samples for each class. However, there are some tasks like detection of fraudulent transactions, for which class imbalance is overwhelming and one of the classes has very low (even less than 10% of the entire data) amount of samples. These tasks often fall under outlier detection. Moreover, there are some scenarios where there may be multiple subsets of the outlier class. In such cases, it should be treated as a multiple outlier type detection scenario. In this article, we have proposed a system that can efficiently handle all the aforementioned problems. We have used stacked autoencoders to extract features and then used an ensemble of probabilistic neural networks to do a majority voting and detect the outliers. Such a system is seen to have a better and reliable performance as compared to the other outlier detection systems in most of the datasets tested upon. It is seen that use of autoencoders clearly enhanced the outlier detection performance.
First Page
161
Last Page
171
DOI
10.1016/j.patcog.2019.01.002
Publication Date
5-1-2019
Recommended Citation
Chakraborty, Debasrita; Narayanan, Vaasudev; and Ghosh, Ashish, "Integration of deep feature extraction and ensemble learning for outlier detection" (2019). Journal Articles. 863.
https://digitalcommons.isical.ac.in/journal-articles/863
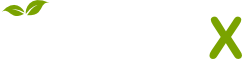
- Citations
- Policy Citations: 2
- Citation Indexes: 91
- Usage
- Abstract Views: 8
- Captures
- Readers: 87