Lattice and imbalance informed multi-label learning
Article Type
Research Article
Publication Title
IEEE Access
Abstract
In a multi-label dataset, an instance is given a single representation across all possible labels. Despite the mutual sharing of instances among the labels, the membership of the instances vary from label to label. This diversifies the intrinsic class geometries of the labels. Multi-label datasets are often found to be class-imbalanced as well. The varying membership of the instances coupled with the imbalance phenomenon gives rise to varying imbalance ratios across the labels. We address these two key aspects in this work, Lattice and Imbalance Informed Multi-label Learning (LIIML) in a two step procedure. Firstly, we obtain the imbalance ratios and the intrinsic positive and negative class lattices of each label. We capitalize on these two information to obtain a dedicated feature set for each label. In the second step, to handle the class-imbalance further, we employ a scheme of imbalance-adaptive misclassification cost across the labels. We have evaluated the competence of the proposed method in a generic as well as class-imbalanced framework. The elaborate empirical study establishes the competence of the proposed method in both the contexts.
First Page
7394
Last Page
7407
DOI
10.1109/ACCESS.2019.2962201
Publication Date
1-1-2020
Recommended Citation
Sadhukhan, Payel and Palit, Sarbani, "Lattice and imbalance informed multi-label learning" (2020). Journal Articles. 536.
https://digitalcommons.isical.ac.in/journal-articles/536
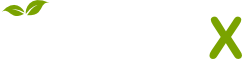
- Citations
- Patent Family Citations: 1
- Policy Citations: 1
- Citation Indexes: 4
- Usage
- Abstract Views: 5
- Captures
- Readers: 8
Comments
Open Access, Gold