Article Type
Research Article
Publication Title
IEEE Journal of Selected Topics in Applied Earth Observations and Remote Sensing
Abstract
Motivation in the use of semisupervised learning method is because of its ability to strategically explore and use abundantly available unlabeled samples along with the limited number of labeled samples, as seen in the remote sensing (RS) imagery. In this direction, the present article proposes a semisupervised classification model with spatial information based self-learning methodology to classify land covers in RS images. The model uses granular neural network (GNN) as the base classifier because of its customizable network architecture that is functionally interpretable and costs less computational complexity. Architecture of GNN is governed by fuzzy if-then rules that are generated from fuzzy granulation of input feature space. We have used an improved spatial neighborhood learning method for better understanding of data distribution in a semisupervised framework. The method collects the information with collaborative opinions of two independent information extraction approaches, i.e., based on mutual neighborhood criteria and class map of unlabeled samples. Superiority of the proposed model with existing methods are established with different RS images in terms of various performance measurement indexes.
First Page
329
Last Page
340
DOI
10.1109/JSTARS.2019.2961985
Publication Date
1-1-2020
Recommended Citation
Kothari, Neeta S.; Meher, Saroj K.; and Panda, Ganapati, "Improved Spatial Information Based Semisupervised Classification of Remote Sensing Images" (2020). Journal Articles. 520.
https://digitalcommons.isical.ac.in/journal-articles/520
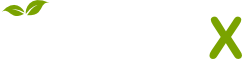
- Citations
- Citation Indexes: 9
- Policy Citations: 1
- Usage
- Downloads: 49
- Abstract Views: 3
- Captures
- Readers: 16
Comments
Open Access, Gold