Local jet pattern: a robust descriptor for texture classification
Article Type
Research Article
Publication Title
Multimedia Tools and Applications
Abstract
Methods based on locally encoded image features have recently become popular for texture classification tasks, particularly in the existence of large intra-class variation due to changes in illumination, scale, and viewpoint. Inspired by the theories of image structure analysis, this work proposes an efficient, simple, yet robust descriptor namely local jet pattern (Ljp) for texture classification. In this approach, a jet space representation of a texture image is computed from a set of derivatives of Gaussian (DtGs) filter responses up to second order, so-called local jet vectors (Ljv), which also satisfy the Scale Space properties. The Ljp is obtained by using the relation of center pixel with its’ local neighborhoods in jet space. Finally, the feature vector of a texture image is formed by concatenating the histogram of Ljp for all elements of Ljv. All DtGs responses up to second order together preserves the intrinsic local image structure, and achieves invariance to scale, rotation, and reflection. This allows us to design a discriminative and robust framework for texture classification. Extensive experiments on five standard texture image databases, employing nearest subspace classifier (Nsc), the proposed descriptor achieves 100%, 99.92%, 99.75%, 99.16%, and 99.65% accuracy for Outex_TC10, Outex_TC12, KTH-TIPS, Brodatz, CUReT, respectively, which are better compared to state-of-the-art methods.
First Page
4783
Last Page
4809
DOI
10.1007/s11042-018-6559-3
Publication Date
2-1-2020
Recommended Citation
Roy, Swalpa Kumar; Chanda, Bhabatosh; Chaudhuri, Bidyut B.; Ghosh, Dipak Kumar; and Dubey, Shiv Ram, "Local jet pattern: a robust descriptor for texture classification" (2020). Journal Articles. 419.
https://digitalcommons.isical.ac.in/journal-articles/419
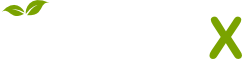
- Citations
- Citation Indexes: 10
- Policy Citations: 1
- Usage
- Abstract Views: 1
- Captures
- Readers: 15
Comments
Open Access, Green