Fast automatic estimation of the number of clusters from the minimum inter-center distance for k-means clustering
Article Type
Research Article
Publication Title
Pattern Recognition Letters
Abstract
Center-based clustering methods like k-Means intend to identify closely packed clusters of data points by respectively finding the centers of each cluster. However, k-Means requires the user to guess the number of clusters, instead of estimating the same on the run. Hence, the incorporation of accurate automatic estimation of the natural number of clusters present in a data set is important to make a clustering method truly unsupervised. For k-Means, the minimum of the pairwise distance between cluster centers decreases as the user-defined number of clusters increases. In this paper, we observe that the last significant reduction occurs just as the user-defined number surpasses the natural number of clusters. Based on this insight, we propose two techniques: the Last Leap (LL) and the Last Major Leap (LML) to estimate the number of clusters for k-Means. Over a number of challenging situations, we show that LL accurately identifies the number of well-separated clusters, whereas LML identifies the number of equal-sized clusters. Any disparity between the values of LL and LML can thus inform a user about the underlying cluster structures present in the data set. The proposed techniques are independent of the size of the data set, making them especially suitable for large data sets. Experiments show that LL and LML perform competitively with the best cluster number estimation techniques while imposing drastically lower computational burden.
First Page
72
Last Page
79
DOI
10.1016/j.patrec.2018.09.003
Publication Date
12-1-2018
Recommended Citation
Gupta, Avisek; Datta, Shounak; and Das, Swagatam, "Fast automatic estimation of the number of clusters from the minimum inter-center distance for k-means clustering" (2018). Journal Articles. 1134.
https://digitalcommons.isical.ac.in/journal-articles/1134
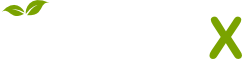
- Citations
- Policy Citations: 1
- Citation Indexes: 34
- Usage
- Abstract Views: 1
- Captures
- Readers: 49