Document Type
Research Article
Publication Title
Genomics
Abstract
Gene expression analysis plays a significant role for providing molecular insights in cancer. Various genetic and epigenetic factors (being dealt under multi-omics) affect gene expression giving rise to cancer phenotypes. A recent growth in understanding of multi-omics seems to provide a resource for integration in interdisciplinary biology since they altogether can draw the comprehensive picture of an organism's developmental and disease biology in cancers. Such large scale multi-omics data can be obtained from public consortium like The Cancer Genome Atlas (TCGA) and several other platforms. Integrating these multi-omics data from varied platforms is still challenging due to high noise and sensitivity of the platforms used. Currently, a robust integrative predictive model to estimate gene expression from these genetic and epigenetic data is lacking. In this study, we have developed a deep learning-based predictive model using Deep Denoising Auto-encoder (DDAE) and Multi-layer Perceptron (MLP) that can quantitatively capture how genetic and epigenetic alterations correlate with directionality of gene expression for liver hepatocellular carcinoma (LIHC). The DDAE used in the study has been trained to extract significant features from the input omics data to estimate the gene expression. These features have then been used for back-propagation learning by the multilayer perceptron for the task of regression and classification. We have benchmarked the proposed model against state-of-the-art regression models. Finally, the deep learning-based integration model has been evaluated for its disease classification capability, where an accuracy of 95.1% has been obtained.
First Page
2833
Last Page
2841
DOI
10.1016/j.ygeno.2020.03.021
Publication Date
7-2020
Recommended Citation
Seal, Dibyendu Bikash; Das, Vivek; Goswami, Saptarsi; and De, Rajat K., "Estimating gene expression from DNA methylation and copy number variation: A deep learning regression model for multi-omics integration" (2020). ISI Best Publications. 56.
https://digitalcommons.isical.ac.in/ibp/56
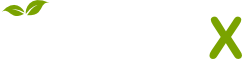
- Citations
- Citation Indexes: 42
- Policy Citations: 1
- Usage
- Downloads: 299
- Abstract Views: 35
- Captures
- Readers: 96
Comments
Open Access, Bronze