Automation of feature engineering for IoT analytics
Document Type
Conference Article
Publication Title
ACM SIGBED Review
Abstract
This paper presents an approach for automation of interpretable feature selection for Internet Of Things Analytics (IoTA) using machine learning (ML) techniques. Authors have conducted a survey over different people involved in different IoTA based application development tasks. The survey reveals that feature selection is the most time consuming and niche skill demanding part of the entire workflow. This paper shows how feature selection is successfully automated without sacrificing the decision making accuracy and thereby reducing the project completion time and cost of hiring expensive resources. Several pattern recognition principles and state of art (SoA) ML techniques are followed to design the overall approach for the proposed automation. Three data sets are considered to establish the proof-of-concept. Experimental results show that the proposed automation is able to reduce the time for feature selection to 2 days instead of 4 - 6 months which would have been required in absence of the automation. This reduction in time is achieved without any sacrifice in the accuracy of the decision making process. Proposed method is also compared against Multi Layer Perceptron (MLP) model as most of the state of the art works on IoTA uses MLP based Deep Learning. Moreover the feature selection method is compared against SoA feature reduction technique namely Principal Component Analysis (PCA) and its variants. The results obtained show that the proposed method is effective.
First Page
24
Last Page
30
DOI
10.1145/3231535.3231538
Publication Date
6-5-2018
Recommended Citation
Banerjee, Snehasis; Chattopadhyay, Tanushyam; Pal, Arpan; and Garain, Utpal, "Automation of feature engineering for IoT analytics" (2018). Conference Articles. 87.
https://digitalcommons.isical.ac.in/conf-articles/87
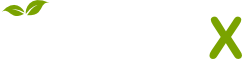
- Citations
- Citation Indexes: 17
- Policy Citations: 1
- Usage
- Abstract Views: 1
- Captures
- Readers: 48
Comments
Open Access, Green